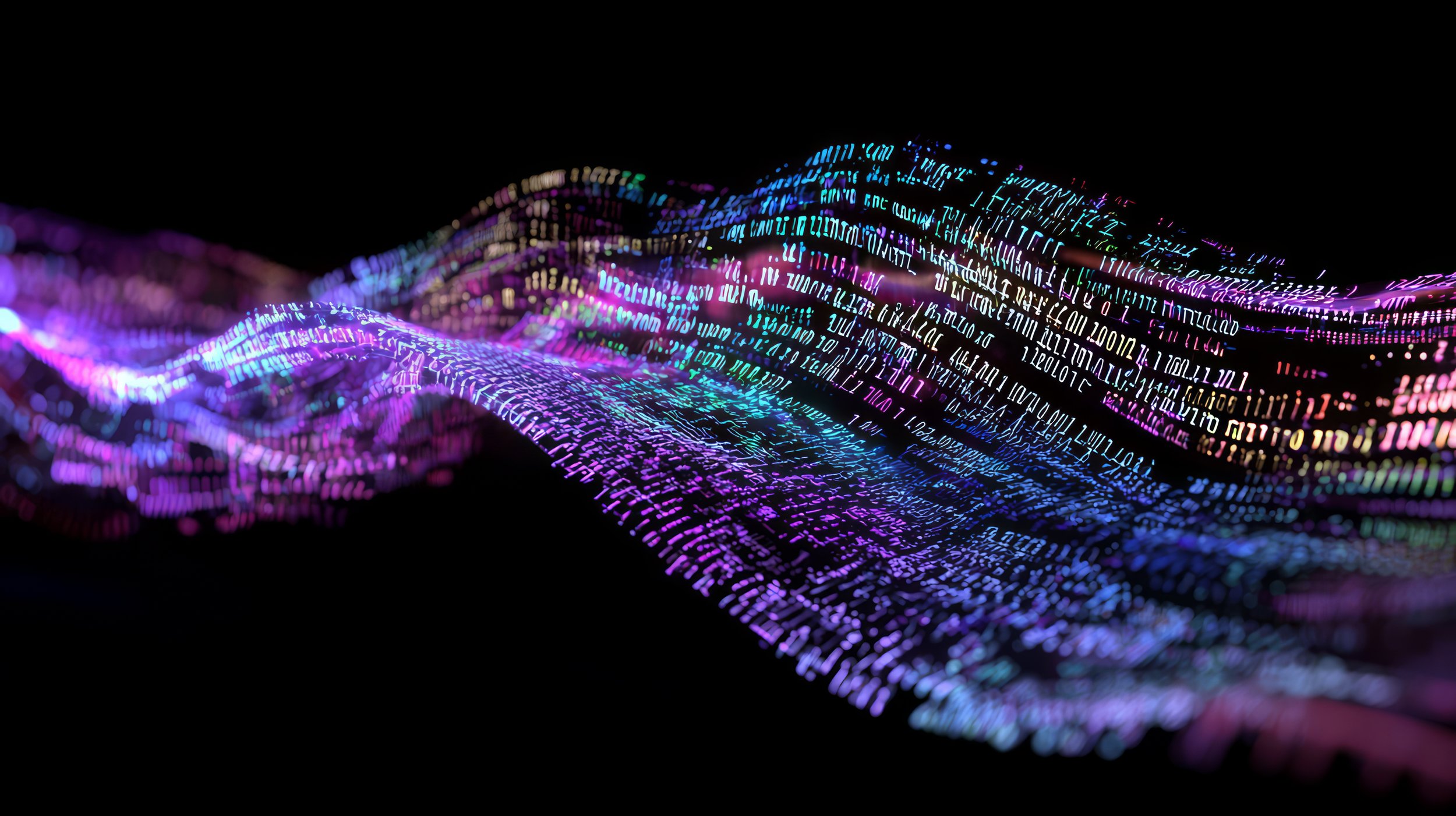
The Large Language Model Lab
The Large Language Model (LLM) Lab is building the future of clinical intelligence. By advancing inference efficiency and developing multi-modal knowledge graphs, we’re making AI more secure, affordable, and precise for real-world medical use.
Leading with Vision and Expertise
Lab Director
Nick Schaub, PhD
Dr. Schaub is an interdisciplinary AI research scientist focusing on applications in biology and medicine. His most recent work, Ask AIthena, is a part of the National AI Research Resource (NAIRR) pilot program. Ask AIthena is a large language model (LLM) research augmented generation (RAG) model that aims to accelerate science by giving AI access to all scientific and medical texts (currently 250 million texts). In addition to his LLM work, Dr. Schaub uses AI for computer vision at scale, using AI models to analyzes 100s of terabytes to petabytes of biological data for drug discovery.
Prior to getting involved in AI, he performed neuroscience and materials research to create materials for nerve regeneration, stimulation, and drug delivery. He holds patents for the use of AI in stem cell biomanufacturing and polymeric drug delivery devices. His current interests are in large scale application of AI to science and medicine, specifically around making data AI accessible to discover links between knowledge in the data and literature.
About Us
The Large Language Model Lab
The LLM Lab is focused on advancing the next generation of medical AI through two core areas: improving inference efficiency and building multi-modal knowledge graphs for medicine and biology. This work aims to reduce costs, enhance security, and increase precision in how AI supports clinical decisions.
LLMs today often require expensive infrastructure and rely on third-party systems to process sensitive health information. Meanwhile, delivering truly effective clinical insights frequently requires the fusion of data from multiple sources—medical literature, patient records, diagnostic images, and more.
The LLM Lab addresses these challenges by:
Developing new metrics and architectures that reduce the computational burden of deploying LLMs, enabling secure, local model deployment without compromising performance.
Building knowledge graphs that intelligently connect research data, clinical records, and medical images to deliver real-time, context-aware insights tailored to each patient.
By pushing the boundaries of LLM development, the lab aims to make AI more accessible, secure, and impactful across the healthcare ecosystem.
Dr. Schaub’s Select Publications
-
Schaub, N. J., and Hotaling, N., “Assessing Efficiency in Artificial Neural Networks,” Applied Sciences, Vol. 13, No. 18, 2023, p. 10286. https://doi.org/10.3390/app131810286.
-
Bajcsy P, Schaub NJ, Majurski M. Designing Trojan Detectors in Neural Networks Using Interactive Simulations. Applied Sciences2021, 11 (4), 1865. https://doi.org/10.3390/app11041865
-
Padi S, Manescu P, Schaub NJ, Hotaling N, Simon C, Bharti K, Bajcsy P. Comparison of Artificial Intelligence Based Approaches to Cell Function Prediction. Informatics in Medicine Unlocked 2020, 18, 100270. https://doi.org/10.1016/j.imu.2019.100270
-
Majurski M, Manescu P, Padi S, Schaub NJ, Hotaling N, Simon C, Bajcsy P. Cell Image Segmentation Using Generative Adversarial Networks, Transfer Learning, and Augmentations. CVPR 2019. https://doi.org/10.1109/CVPRW.2019.00145
-
Moore, J., et al. “OME-Zarr: A Cloud-Optimized Bioimaging File Format with International Community Support,” Histochemistry and Cell Biology, 2023. https://doi.org/10.1007/s00418-023-02209-1
-
Ishaq, N., Hotaling, N., and Schaub, N., “Theia: Bleed-Through Estimation With Convolutional Neural Networks,” presented at the Proceedings of the IEEE/CVF Conference on Computer Vision and Pattern Recognition, 2023. https://doi.org/10.1109/CVPRW59228.2023.00447
-
Goyal, V., Schaub, N. J., Voss, T. C., and Hotaling, N., “Unbiased Image Segmentation Assessment Toolkit for Quantitative Differentiation of State-of-the-Art Algorithms and Pipelines,” BMC Bioinformatics, Vol. 24, No. 1, 2023, p. 388. https://doi.org/10.1186/s12859-023-05486-8.
-
Florczyk, S., Hotaling, N., Simon, M., Chalfoun, J., Horenberg, A., Schaub, N., Wang, D., Szczypiński, P., DeFelice, V., Bajcsy, P., and Simon, C. “Measuring Dimensionality of Cell-Scaffold Contacts of Primary Human Bone Marrow Stromal Cells Cultured on Electrospun Fiber Scaffolds.” Journal of Biomedical Materials Research. Part A, 2022. https://doi.org/10.1002/jbm.a.37449.
-
Schaub, N. J.*, Hotaling, N.*, Manescu, P., Padi, S., Wan, Q., Sharma, R., George, A., Chalfoun, J., Simon, M., Ouladi, M., Carl G. Simon, J., Bajcsy, P., and Bharti, K. “Deep Learning Predicts Function of Live Retinal Pigment Epithelium from Quantitative Microscopy.” The Journal of Clinical Investigation, Vol. 2, No. 130, 2019, pp. 1010–1023. https://doi.org/10.1172/JCI131187.