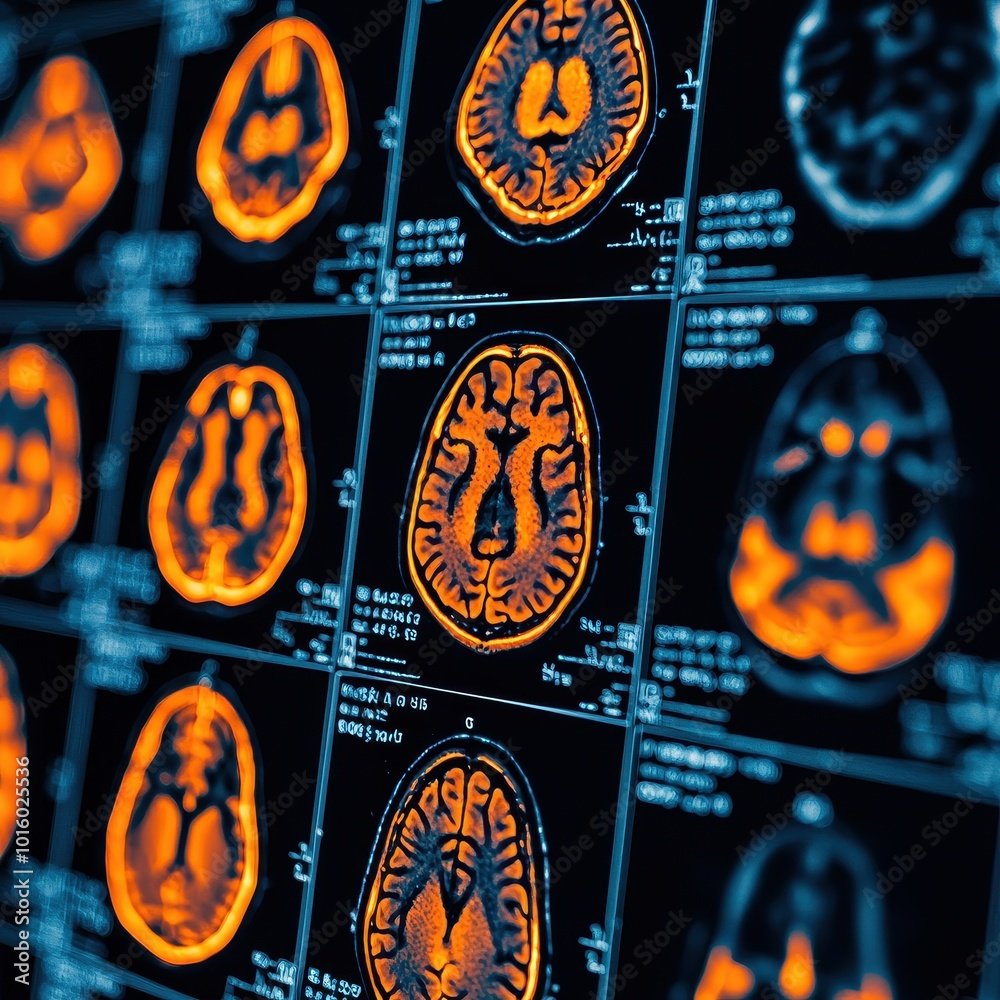
IAMAI Center
At NovaGen’s IAMAI Center, we are advancing the frontier of medical AI. Our vision is to pioneer collaborative, cutting-edge AI methods that uncover disease processes, deepen medical understanding, and improve patient outcomes. Through rigorous research and strategic partnerships, IAMAI empowers physicians and AI researchers to transform the future of healthcare.
The IAMAI Imaging AI Lab
At the IAMAI Center, our Imaging AI lab is dedicated to advancing the use of computer vision and artificial intelligence to interpret and analyze medical images and complex health data. We develop intelligent systems that support clinical decision-making and medical discovery through the following focus areas:
AI Modeling & Medical Imaging – Creating intelligent models for analyzing diagnostic images.
Multi-Modal AI – Integrating diverse data sources for a more complete clinical picture.
Longitudinal AI – Tracking patient data over time to uncover patterns and predict outcomes.
Federated Learning – Enabling AI development across distributed datasets while preserving privacy.
Medical Image Processing – Enhancing and extracting insights from radiologic and pathology images.
Leadership Team
Lorem Ipsum is a seasoned professional with extensive experience in the fields of lorem, ipsum, and dolor sit amet. With a background in consectetur adipiscing elit, Lorem has led numerous projects focused on delivering impactful, scalable solutions that bridge innovation and strategy.
Over the course of a distinguished career, Lorem has held leadership roles across various sectors, including technology, healthcare, and non-profit ventures. Known for a commitment to excellence and a collaborative approach, Lorem continues to drive meaningful progress in the ever-evolving landscape of lorem ipsum research and development.
Craig Jones Ph.D
Lab Director
The IAMAI Large Language Model Lab
At the IAMAI Center, Dr. Nick Schaub’s Large Language Model (LLM) Lab is focused on advancing the next generation of medical AI through two core areas: improving inference efficiency and building multi-modal knowledge graphs for medicine and biology. This work aims to reduce costs, enhance security, and increase precision in how AI supports clinical decisions.
LLMs today often require expensive infrastructure and rely on third-party systems to process sensitive health information. Meanwhile, delivering truly effective clinical insights frequently requires the fusion of data from multiple sources—medical literature, patient records, diagnostic images, and more.
The IAMAI LLM Lab addresses these challenges by:
Developing new metrics and architectures that reduce the computational burden of deploying LLMs, enabling secure, local model deployment without compromising performance.
Building knowledge graphs that intelligently connect research data, clinical records, and medical images to deliver real-time, context-aware insights tailored to each patient.
By pushing the boundaries of LLM development, the lab aims to make AI more accessible, secure, and impactful across the healthcare ecosystem.
Nick Schaub Ph.D
Lab Director
Leadership Team
Dr. Nick Schaub, Ph.D., is an interdisciplinary AI research scientist, focusing on applications in biology and medicine. His most recent work, Ask AIthena, is a part of the National AI Research Resource (NAIRR) pilot program. Ask AIthena is a large language model (LLM) research augmented generation (RAG) model that aims to accelerate science by giving AI access to all scientific and medical texts (currently 250 million texts). In addition to his LLM work, Dr. Schaub uses AI for computer vision at scale, using AI models to analyzes 100s of terrabytes to petabytes of biological data for drug discovery.
Prior to getting involved in AI, he performed neuroscience and materials research to create materials for nerve regeneration, stimulation, and drug delivery. He holds patents for the use of AI in stem cell biomanufacturing and polymeric drug delivery devices. His current interests are in large scale application of AI to science and medicine, specifically around making data AI accessible to discover links between knowledge in the data and literature.